Hiring Data Scientists: Do I Need One and How to Find the Best?
XEC Recruitment
In today’s data-driven world, businesses are increasingly looking to leverage the power of data to gain insights and drive decision-making. One key role in this endeavor is that of a data scientist. This article will explore whether you need a data scientist, what they can do for your business, the potential for automation without one, their role within an AI team, and how to find a top-notch data scientist.
Do I Need a Data Scientist?
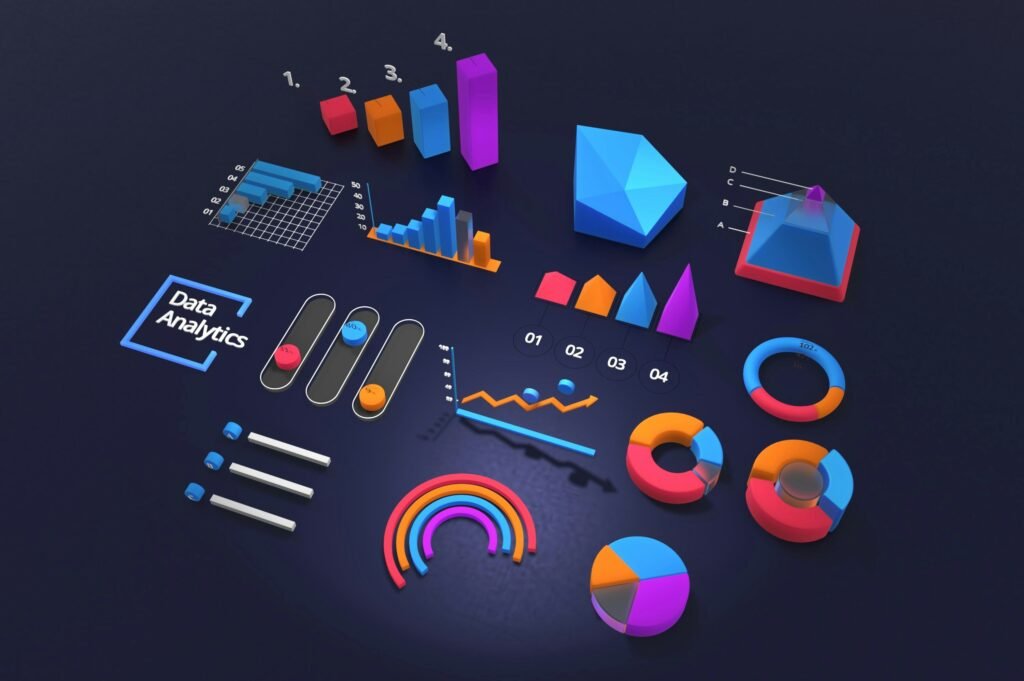
Assessing Your Business Needs
The first step in determining whether you need a data scientist is to assess your business needs and goals. Data scientists are valuable assets for companies looking to analyze large volumes of data, build predictive models, and derive actionable insights. If your business handles vast amounts of data and seeks to harness this data for strategic advantage, hiring a data scientist could be essential.
Key Considerations:
- Data Volume: High data volume can be overwhelming without a dedicated expert.
- Complexity of Analysis: Advanced statistical and machine learning techniques often require specialized skills.
- Strategic Objectives: If your business aims to become more data-driven in its decision-making processes.
Common Indicators You Need a Data Scientist
- Data Overload: If your business is generating more data than you can handle or interpret.
- Complex Analysis: When your data analysis needs surpass basic statistical techniques and require advanced modeling.
- Predictive Insights: If you need to forecast trends, customer behavior, or operational efficiencies.
- Data-Driven Decision Making: When decisions need to be backed by in-depth data analysis and insights.
What Can Data Scientists Do?
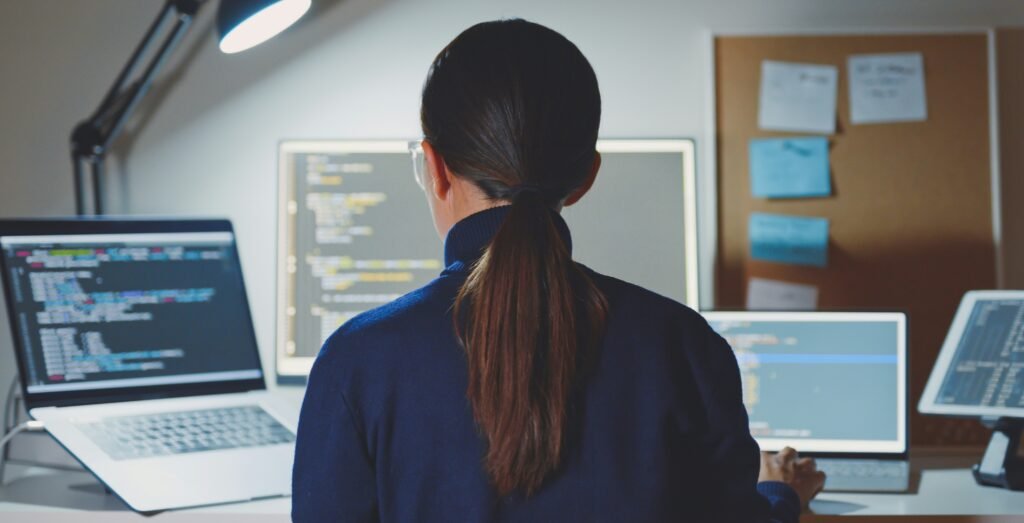
Key Responsibilities and Skills
Data scientists possess a unique blend of skills in statistics, programming, and domain expertise. Their key responsibilities typically include:
- Data Cleaning and Preparation: Ensuring data is accurate and ready for analysis. This involves dealing with missing values, outliers, and ensuring consistency across data sets.
- Exploratory Data Analysis (EDA): Identifying patterns and insights from raw data. EDA helps in understanding the main characteristics of the data.
- Machine Learning and Predictive Modeling: Building algorithms to predict future trends or behaviors. This involves selecting the right model, training it on data, and validating its performance.
- Data Visualization: Creating visual representations of data to communicate findings clearly. Tools like Tableau, Power BI, or custom visualizations in Python/R can be used.
- Reporting and Communication: Translating complex data insights into actionable recommendations for stakeholders. Effective communication ensures that the insights are understood and can be acted upon.
Business Applications
Data scientists can drive significant value across various business functions, such as:
- Marketing: Personalizing marketing campaigns based on customer segmentation. Data scientists can help identify customer segments and target them with tailored marketing strategies.
- Sales: Predicting customer churn and identifying upsell opportunities. Advanced models can forecast which customers are likely to leave and which might be interested in additional products.
- Operations: Optimizing supply chain logistics and inventory management. Data scientists can analyze operational data to find efficiencies and reduce costs.
- Finance: Detecting fraud and improving risk management. Predictive models can identify suspicious transactions and assess financial risks.
Can I Do Automation on My Own?
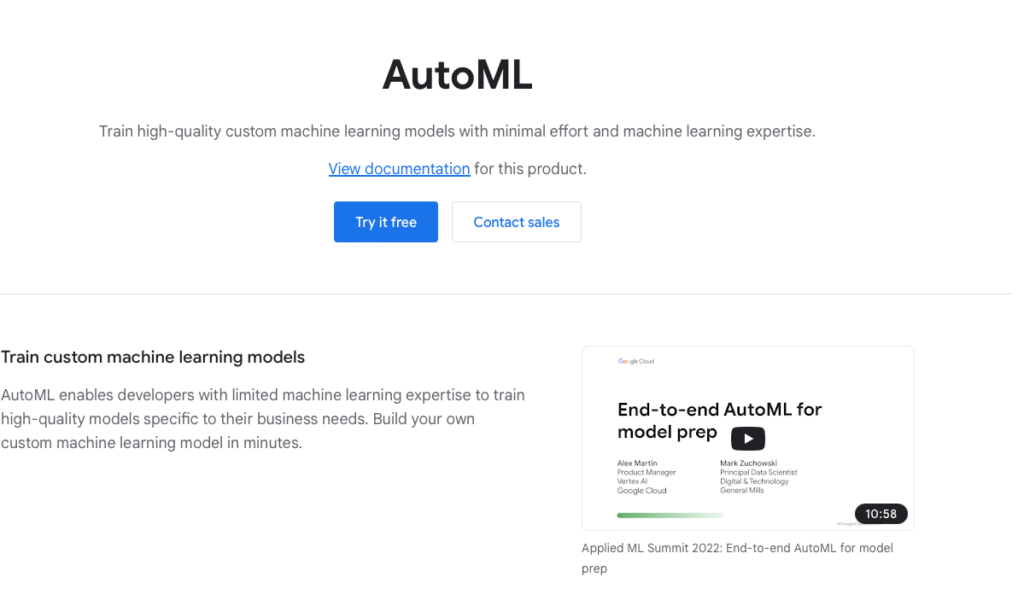
Understanding the Scope of Automation
Automation tools have become increasingly sophisticated, allowing businesses to perform many data-related tasks without needing a full-time data scientist. Tools like automated machine learning (AutoML) platforms can handle data preprocessing, model selection, and hyperparameter tuning.
Popular Tools:
- AutoML: Platforms like Google AutoML and H2O.ai automate many steps in the machine learning pipeline.
- Data Wrangling Tools: Tools like Trifacta and Alteryx help in cleaning and preparing data for analysis.
- Business Intelligence (BI) Tools: Tableau, Power BI, and Looker can automate data visualization and reporting.
Limitations of Automation
While automation can handle routine data tasks, it has limitations:
- Complex Problem Solving: Automated tools may struggle with nuanced, complex problems that require human intuition and domain knowledge.
- Customization: Automation tools may lack the flexibility to tailor models to specific business needs.
- Interpretability: Data scientists can provide deeper insights and interpret model outcomes in the context of the business, something automation tools might not fully achieve.
When Automation Suffices
Automation can be sufficient for businesses with straightforward data needs, such as basic reporting and simple predictive analytics. However, for more advanced analytics and strategic data initiatives, a data scientist’s expertise is invaluable.
How Do Data Scientists Fit Into an AI Team?
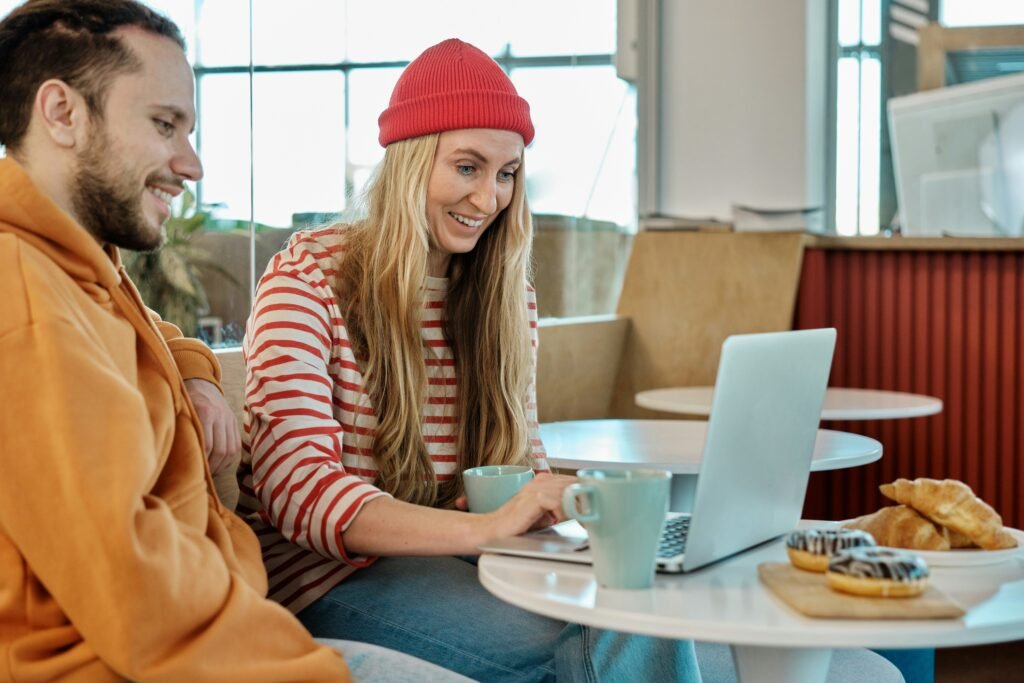
The Role of Data Scientists in AI Teams
Data scientists are integral to AI teams, contributing their expertise in data analysis and modeling to support AI development. They work closely with other roles, such as:
- Data Engineers: Who manage data pipelines and infrastructure. Data engineers ensure that data scientists have access to clean and reliable data.
- Machine Learning Engineers: Who focus on deploying machine learning models into production. They work on integrating the models developed by data scientists into the company’s software systems.
- Business Analysts: Who translate business requirements into data projects. They ensure that the data science projects align with business goals.
- AI Researchers: Who develop cutting-edge algorithms and approaches. These researchers push the boundaries of what is possible with AI and machine learning.
Collaborative Efforts
In an AI team, data scientists collaborate to:
- Define Objectives: Work with stakeholders to understand business goals and define data objectives. This ensures that data projects are aligned with business strategy.
- Data Strategy: Develop strategies for data collection, analysis, and utilization. This involves setting up data governance and ensuring data quality.
- Model Development: Build and refine machine learning models to solve specific business problems. This includes selecting appropriate algorithms and fine-tuning models.
- Deployment and Monitoring: Ensure models are effectively deployed and monitored for performance. Continuous monitoring helps in maintaining the accuracy and relevance of models over time.
How to Find a Good Data Scientist
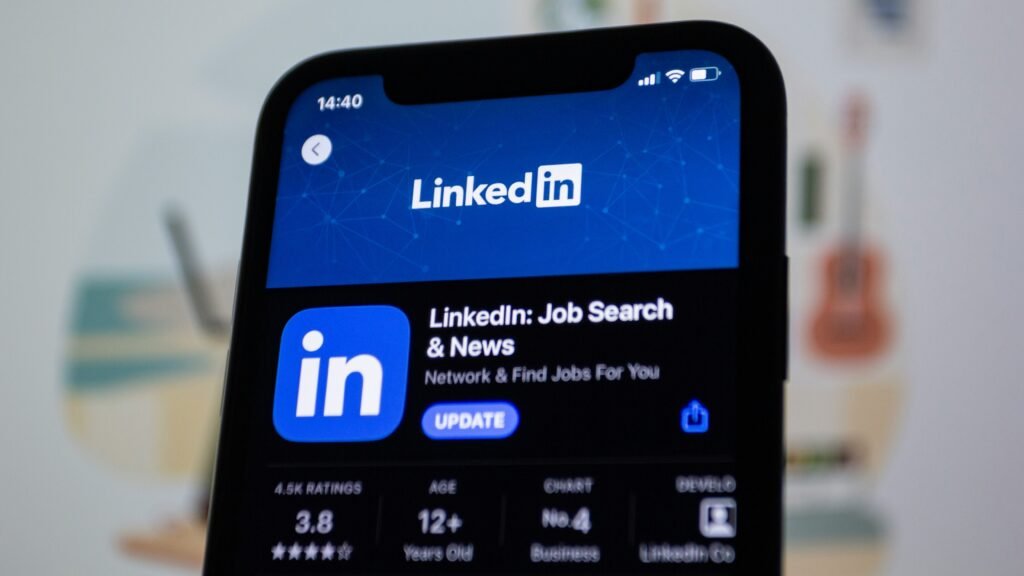
Defining Your Needs
Before starting the hiring process, clearly define your needs and the specific skills required for the role. Consider the following:
- Technical Skills: Proficiency in programming languages (Python, R), machine learning frameworks (TensorFlow, PyTorch), and data visualization tools (Tableau, Power BI).
- Domain Expertise: Experience in your industry or relevant domain knowledge.
- Soft Skills: Strong communication, problem-solving, and collaboration skills.
Sourcing Candidates
- Job Boards and Networks: Utilize job boards like LinkedIn, Glassdoor, and specialized data science platforms such as Kaggle.
- University Partnerships: Partner with universities to access fresh talent from data science programs.
- Recruitment Agencies: Leverage AI recruitment agencies like XEC Recruitment, which specialize in sourcing top-tier AI and data science talent.
Evaluating Candidates
- Technical Assessments: Conduct coding tests, data analysis challenges, and machine learning tasks to evaluate technical proficiency.
- Portfolio Review: Review candidates’ previous projects and contributions to open-source projects or competitions.
- Behavioral Interviews: Assess cultural fit and soft skills through behavioral interviews and scenario-based questions.
Why Use an AI Recruitment Agency
Partnering with a specialized AI recruitment agency like XEC Recruitment can significantly streamline the hiring process. Their expertise in the field ensures:
- Tailored Job Descriptions: Crafting precise job descriptions that attract the right candidates.
- Cultural Fit: Understanding your company culture to find candidates who align well with your organization.
- Access to Passive Candidates: Tapping into a broader pool of candidates, including those not actively seeking new opportunities.
- Efficient Screening: Utilizing advanced tools and methodologies to efficiently screen and vet candidates.
Conclusion
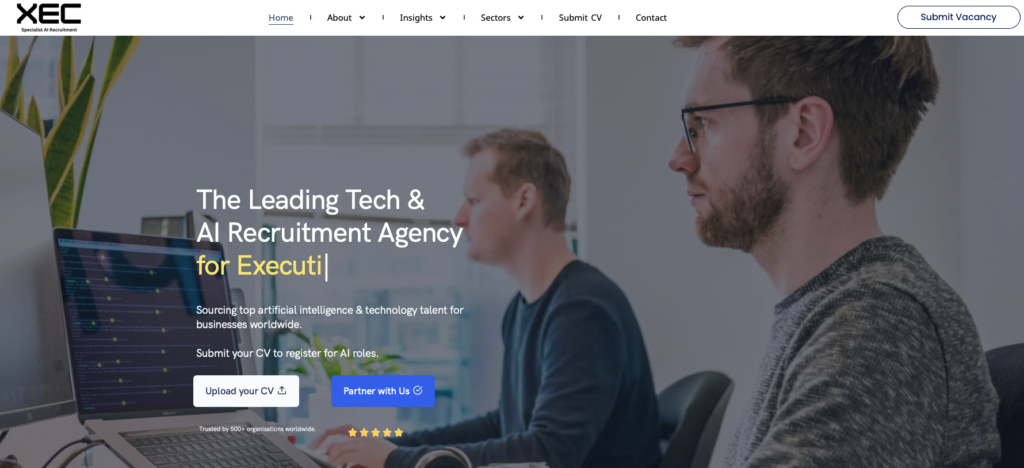
Hiring a data scientist can provide your business with the expertise needed to harness the power of data and drive strategic initiatives. While automation tools can handle some tasks, the complex problem-solving and customized insights provided by a skilled data scientist are invaluable. By clearly defining your needs, leveraging multiple recruitment channels, and considering the benefits of partnering with an AI recruitment agency like XEC Recruitment, you can attract and retain top-tier data science talent to propel your business forward.